Poster Presentations
Room Layout
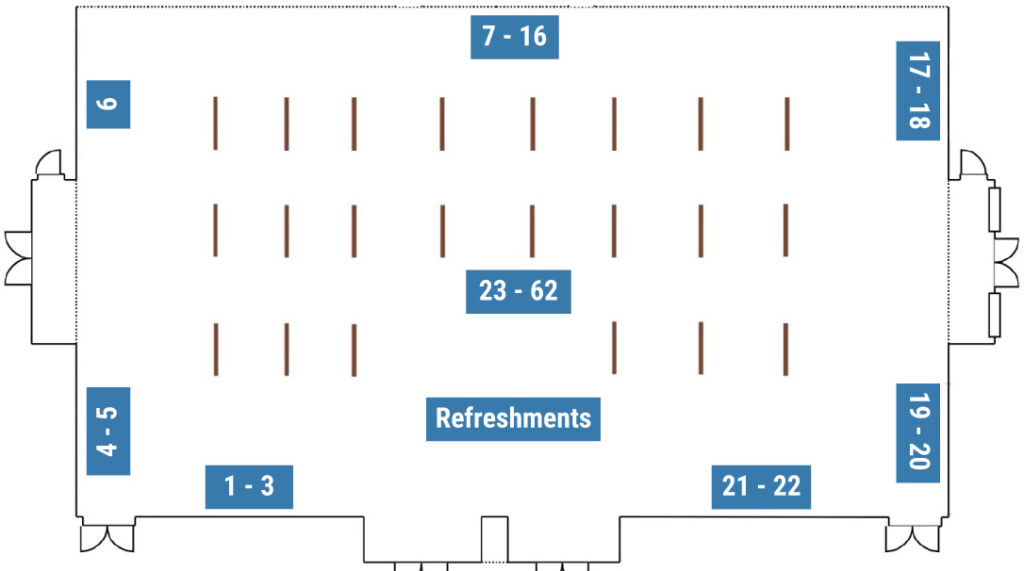
1 – Improving the Magnetic Permeability of Composite Materials to Enhance Wireless Power Transfer System Performance for Transportation
Xiuhu Sun (UNC Charlotte)
Wireless power transfer (WPT) enables charging without human intervention and serves as critical infrastructure for electric vehicles in the transportation sector. In the transportation WPT system, magnetic composite materials, such as magnetic concrete consisting of magnetic particles and cement, can significantly improve the efficiency and overall performance of the system by enhancing electromagnetic induction. However, the high cost of these materials poses a challenge, particularly for large-scale applications like charging highways and rails, which affects their economic feasibility. This study aims to optimize the geometry of magnetic concrete to improve its coupling coefficient while reducing material usage. Additionally, by applying electromagnetic pulses, the magnetic material can be redistributed to increase its effective magnetic permeability. Ultimately, these approaches will improve the efficiency and performance of WPT systems while saving materials and enhancing economic viability.
2 – Variability of Plant-produced High Recycled Content Asphalt Mixtures within North Carolina
Gabriel Macedo Duarte (NC State University)
The incorporation of recycled asphalt material (RAM) into asphalt mixtures is a widely adopted technique in the asphalt paving industry as it reduces the demand for raw materials such as virgin aggregate and asphalt binder, thereby preserving natural resources, decreasing landfill waste from construction debris, and lowering costs. State agencies limit the quantity of recycled asphalt pavement (RAP) and recycled asphalt shingles (RAS) in asphalt mixtures owing in part to concerns about variability in performance. The variability of RAM stockpile properties within a state can lead to inconsistencies in the properties and performance of asphalt mixtures. This study aimed to characterize the properties of RAM materials within stockpiles over time and across multiple asphalt plants in North Carolina. It also evaluated the variability in performance of plant-produced high recycled content mixtures in the state and investigated the potential causes for this variability. Asphalt mixtures and their constituent materials were acquired and characterized from four asphalt plants on two different dates. The results showed that while RAM properties were generally consistent within stockpiles over time, significant variations in binder properties and asphalt content were found across different plants within the state. The majority of the mixtures evaluated showed consistent rutting and cracking performance. Variability in cracking performance across plants could be linked to the properties of the recycled and virgin binders. The differences in cracking and rutting performance between the first and second samples acquired from a given plant closely mirrored asphalt content differences.
3 – Characterize Shared E-scooter Travel Patterns using Comprehensive Travel Data: A Case Study in Charlotte, NC
Yuqiu Yuan (UNC Charlotte)
Shared e-scooters have gained popularity as a micro-mobility option in urban areas and have the potential to improve transportation convenience and efficiency by replacing walking, and to enhance energy and environmental benefit by saving car driving trips. This study aims to process and analyze the e-scooter data collected from Charlotte, NC, to understand the detailed e-scooter user travel and trip-level activity through a series of descriptive and temporal-spatial analyses. With that, Charlotte’s micromobility travel patterns and insights can be identified. Furthermore, e-scooter data has been spatially aligned and analyzed with a variety of travel-related data, such as land use, demographic census block groups, and public transit data at different resolution levels (e.g., micro- and macro-levels) to further explore the e-scooter use cases and the role of e-scooters in first-and-last-mile (FLM) transport in Charlotte. The micro-level analysis focuses on the ride pickup or drop-off (PUDO) points, transit accessibility, and land use characteristics. The micro-level e-scooter trips were further aggregated into the census block groups in a macro-level analysis, which provides insights into e-scooter’s travel demand patterns, such as origin-destination matrix, production-attraction of census block groups, and accessibility to e-scooter pickup and drop-off locations. The analyses offer unique sociodemographic perspectives on the intricate dynamics of e-scooter mobility in the Charlotte metropolitan area, which have the potential to be applied to enhance urban transportation services, such as transit accessibility, operations of microtransit and e-bikes. The study was supported by NCDOT Technical Assistance Request Project: TA-2024-06 Charlotte Scooter Data Processing and Travel Pattern Analysis.
4 – A Comparative Analysis of Machine Learning-Based Intrusion Detection Systems for Intra-Vehicle Communications in Connected and Autonomous Vehicles (CAVs) in Rural Areas
Mahsa Tavasoli (NC A&T University)
This paper presents an in-depth comparative analysis of ML-based Intrusion Detection Systems (IDS) for securing CAN bus communications in CAVs operating in rural environments. We evaluated models including Random Forest, SVM, Decision Tree, and LSTM for their ability to detect and classify anomalies within CAN bus communications by utilizing both historical data and real-time inputs. Each model exhibited distinct strengths and limitations in addressing the unique cybersecurity needs of rural CAV systems. The LSTM model achieved the highest detection accuracy at 98.14%, making it particularly effective for identifying time-dependent anomalies, such as repeated communication disruptions. However, the model’s extended training duration (36.33 seconds) limits its practicality for real-time applications. In contrast, the Decision Tree model demonstrated the fastest training time (0.02 seconds) and the smallest model size (0.02 MB), making it well-suited for resource-constrained settings where rapid deployment is crucial. Random Forest provided a balanced approach with an accuracy of 97.44% and moderate computational demand, while SVM presented a compromise between complexity and accuracy, ideal for environments with moderate detection needs. Further optimization of the Random Forest model through hyperparameter tuning enhanced its accuracy to 98.94% and reduced the model size from 1.29 MB to 0.52 MB, making it more efficient for resource-limited rural deployments. Our findings underscore the feasibility of deploying ML-based IDS for strengthening CAV cybersecurity in rural regions. Each model’s performance in terms of accuracy, training time, and model size supports the development of adaptable IDS frameworks tailored to the unique challenges of rural vehicular communication networks.
5 – Modified T-FAST Test for Quantifying the Risk of ASR in Hardened Concrete
Aline Cristiane Kumpfer Nascimento (NC State University)
The alkali-silica reaction (ASR) is a significant durability issue in concrete structures. It occurs when alkali-rich pore solutions react with reactive siliceous aggregates, leading to extensive cracking, which often necessitates costly repairs or complete replacement of the affected structures. The most reliable ASR testing method, ASTM C1293-20a (the Concrete Prism Test, CPT), can take up to two years to produce results. To accelerate ASR assessment, faster methods like ASTM C1260 (the Mortar Bar Test) are commonly used but often produce unreliable results. As a response, the Turner-Fairbank Alkali-Silica Reactivity Test (T-FAST) has shown potential as a faster and more accurate ASR testing method, delivering results within 21 days. Unlike traditional methods that rely on expansion measurements, T-FAST assesses aggregate chemical reactivity by measuring ion leaching in sodium hydroxide solutions. T-FAST has demonstrated good agreement with both ASTM C1293-20a and field expansion data. However, a reliable test for concrete samples is still needed. This study aimed to modify the T-FAST protocol to evaluate concrete mixtures, not just aggregates. It investigated variables like temperature (55°C and 80°C), immersion solution composition, and test duration, assessing their influence on the Reactivity Index (RI) of crushed concrete samples. Results from five aggregates tested with the modified T-FAST protocol were compared with 1-year ASTM C1293-20a expansion data. The findings showed a good correlation with the 0.45 RI threshold, indicating suitability for use with crushed concrete samples.
6 – Using Color Measurements to Assess Reclaimed Asphalt Pavement (RAP) Degree of Activity
Rafaella Fonseca da Costa (NC State University)
High content Reclaimed Asphalt Pavement (RAP) mixtures pose a challenge due to uncertainty regarding the activity level of RAP binder during production. This activity level, termed Degree of Activity (DoA), influences the integration of RAP binder into the virgin binder matrix of asphalt mixtures. This study proposes a practical method for measuring DoA using Light Intensity (LI) measurements obtained from the Asphalt Compatibility Tester (ACT). Since darker colors reflect less light, it is hypothesized that LI decreases as the activation level of RAP increases. Thus, LI can provide a quantitative measure associated with DoA for RAP samples. Furthermore, this study compares DoA measurements obtained using the ACT with those obtained using a method associating DoA with Indirect Tensile Strength (ITS) of 100 percent RAP specimens. The two methods showed similar trends, but their measurements occasionally diverged. Both methods demonstrated an increase in DoA as temperatures rose from 100°C to 155°C, with DoA values exceeding 70% at standard production temperatures (140°C–155°C) for hot asphalt mixtures. The ACT-based DoA measurement approach holds promise for improving material selection and the design of recycled asphalt mixtures. However, further validation with a broader range of RAP sources is required to confirm its reliability and applicability across varying material sources.
7 – Identification and Prioritization of Vulnerable Roadway Segments for Proactive Resilience Planning and Response
Matheus Jesus Ribeiro Araújo (NC State University)
Flooding caused by tropical storms, hurricanes, and other weather events can significantly damage pavement infrastructure. Some of the most common issues reported by the North Carolina Department of Transportation are usually associated with immediate damage or long-term effects including failures caused by erosion and weakened bases and subgrade. In addition, infrastructure issues related to these events, such as embankment failures, landslides, and damaged bridges and culverts, significantly impact mobility. It can require substantial resources for rebuilding or rehabilitating the road network and recovering the lost mobility. The main objective of this project is to identify the characteristics that contribute to the vulnerability of roads due to flooding and to identify features that may play a role in improving pavement resilience due to repeated or more severe inundation. Initially, sections expected to be recurrently flooded will be identified through data sources such as the Roadway Inundation Tool (RIT, software that uses LiDAR data to predict riverine and coastal flooding within different recurrence time intervals), official reports, division information, and road closure records. Subsequently, 1) sites reported as damaged after hurricanes, tropical storms, and other weather events and 2) sections predicted to be frequently flooded will be inspected to evaluate the main factors associated with the damage and possible continued effects. Ultimately, the project aims to analyze the relationship between flood-induced pavement deterioration and the common features and characteristics of pavements and roadway infrastructure that contribute to less resilient roadway segments, providing recommendations to support the development of more durable pavement infrastructure.
8 – Designing Artificial Intelligence Tools for Human-Like Automated Driving
Michael Azage (NC A&T University)
Recent advancements in autonomous driving aim to replicate human decision-making, promising enhancements in safety and user acceptance. Accurately modeling the intricate and adaptive nature of human road navigation remains a significant challenge. This research introduces a practical control system designed and implemented to learn from expert human driving behavior using LSTM networks for predictive tasks. The system processes historical buffered data, and current sensor to estimate axle torque and deceleration values. Experimental validation results based on sensor data from an automated Chevy Bolt EUV have shown the system’s predictive accuracy aligns well with ground truth measurements. Furthermore, the system effectively filters out additive noise with zero mean from both current and predicted chunked pitch values, enhancing its noise resistance. Statistical analyses, including measures of central tendency, coefficient of correlation, mean absolute error, and probability density plots, confirm the system’s capability to emulate human-like driving decisions. This approach improves the predictive accuracy and noise resilience of autonomous driving systems, enhancing overall driving safety, comfort, and efficiency by closely mimicking human responsiveness on the road. Additionally, the system enables practical application in real road scenarios with steep inclines or declines by predicting pitch values ahead and adjusting torque and braking accordingly. This behavior correlates with human driver responses, ensuring the autonomous vehicle maintains appropriate propulsion and braking for varying road conditions, ultimately advancing the practicality and reliability of autonomous driving.
9 – Evalute Lane Centering Assist System in Work Zones
Abhinav Sharma (NC State University)
Lane Centering Assist (LCA) systems rely significantly on clear and well-maintained lane markings for reliable operation. In work zones, where inconsistent or worn markings are common, LCA is very likely to malfunction, leading to potential safety hazards. The objective of this research is to capture the potential malfunctions of LCA in work zones and evaluate its safety impacts. To this end, we equip an LCA-enabled vehicle with a multi-sensor system and drive it through work zones with potentially confusing markings to test the LCA performance. The multi-sensor system consists of a centimeter-level GPS, a LiDAR, and three cameras, which produce four datasets, including the subject vehicle’s trajectory, trajectories of surrounding vehicles, a 3D representation of the site environment (including geometrics, lane markings, cones), and video footage of the driving environment. With the data collected, we evaluate the LCA performance. The performance metrics include vehicle’s lateral deviation, yaw rate, and maximum acceleration, which will collectively capture any drift or steering deviations in response to confusing markings. The research outcomes will help government agencies to understand the effects of LCA malfunctions and develop safety enhancement strategies, such as new methods to remove unwanted markings in work zones.
10 – Project OVAL (On-campus Vehicle Autonomous Launch)
Jimin Yu, Cole Malinchock, Jack Elia, Pratik Thapa, and Suchir Madap (NC State University)
Project OVAL aims to deliver a versatile autonomous service for university and business campuses across the United States. OVAL is a compact, self-driving vehicle measuring approximately 2 feet in height, 3 feet in length, and 1.5 feet in width, designed specifically for campus environments. It is capable of multiple functionalities. This includes campus navigation and additional accommodations to provide student safety and assist those with disabilities. Additionally, OVAL can transport packages, food, books, etc. To provide these functions it is equipped with a locker system that can only be opened through QR code scans or a unique numeric code, a camera with an emergency button for security personnel to monitor during emergencies, an on-board first aid/CPR kit, and a touchscreen display and speaker to assist users with directions or other campus information. With its flexible design and accessible technology, OVAL has limitless potential to enhance campus life and meet the needs of students, faculty, and staff. Image segmentation is utilized to identify traversable paths and avoid any obstacles to perform autonomous navigation. This, along with GPS data, allows the vehicle to find the correct path to its desired destination. Large Language Models are also employed in human-computer interaction to enable real-time conversation and clear communication, helping to effectively address users’ needs.This could include warning about oncoming traffic when crossing a road and providing directions to a lost student.
11 – Navigating the Truck Parking Challenge: A Comprehensive Review of Strategies, Emerging Technologies, and Future Directions
Nana Duah (NC A&T University)
Freight transportation systems play a vital role in the economy of the United States, making adequate truck parking essential for safe and efficient operations. However, a significant gap between truck parking demand and supply has led to challenges including road safety risks, regulatory non-compliance, operational inefficiencies, and environmental impacts. Despite their importance, comprehensive reviews of potential solutions for truck parking are limited. This study addresses this knowledge gap by reviewing the current truck parking management approaches, focusing on intelligent truck parking information systems, data prediction models, and truck pattern analysis. The analysis encompasses various technological solutions, including the sensing infrastructure, GPS technology, and information dissemination methods. The review also examines emerging solutions in smart parking systems and the potential adoption of these solutions to address truck parking challenges. Furthermore, the current challenges in the truck parking problem, the possible solutions, and future scopes in its implementation are also presented.
12 – A Hierarchical Deep Reinforcement Learning Based Approach for Robust Decision-Making of Autonomous Vehicles at Unsignalized Intersections in Rural Communities
Miressa Mamo (NC A&T University)
In rural and suburban areas, unsignalized intersections are frequently encountered, and maneuvering at these intersections poses a significant challenge for autonomous vehicles due to the complex interactions among traffic participants and the associated risks. This informs the need for robust decision-making system which can ensure the safety of autonomous vehicles. Traditional rule-based methods have been investigated to address the problem. These methods provide reliable decision-making at intersections in simple scenarios. However, in complex intersections with numerous participants, accounting for all possible rules becomes nearly impossible. In contrast, deep reinforcement learning techniques outperform rule-based methods by covering large observation spaces and eliminating the need for complete knowledge of the environment. In this works, a hierarchical reinforcement learning-based decision-making architecture based on Gated Recurrent Unit (GRU) and Double Deep Q-Networks (DDQN) is proposed for robust intersection crossing. The proposed technique consists of high-level layer for making decisions and low-level layer for determining an optimal steering to traverse a desired route while considering other traffic users. This approach reduces computational complexity by using a hybrid-reward mechanism and decomposing tasks into two subtasks for more efficient learning. A GRU is used at the input to capture and retain history of observations over time, enabling the network to recognize and mitigate the effects of observation noise. The proposed method is tested in the high-fidelity CARLA simulator, utilizing realistic driving environments and vehicle dynamics to evaluate the robustness of our approach.
13 – A robust AI-enabled information fusion for object detection and scene understanding in rural communities
Tekalign Mengistu (NC A&T University)
Abstract: This work presents a robust fusion approach integrating camera and radar data aimed at enhancing scene understanding of automated driving. The proposed method leverages the complementary strengths of both sensing modalities while addressing the challenges of computational efficiency and performance during adverse weather conditions. By combining high-resolution visual data from cameras with precise depth and velocity information from radar, this approach enables a robust understanding of both static and dynamic objects within the environment. Furthermore, the method presents an attention-based fusion architecture aimed at reducing computational overhead by automatically identifying and selecting sparse features from radar data. Focusing in rural areas, experimental results demonstrate the proposed methods effectiveness and efficiency, showcasing its potential for understanding challenging scenes caused by adverse weather, thus enabling safe and efficient navigation.
14 – Mobile Health Clinic Routing Optimization Using Synchronization Constraints and Comparative Analysis of Meta-Heuristics
Sam Jotham Sutharson (NC A&T University)
Mobile health clinics (MHCs) bridge the gap between traditional hospital visits and home-based healthcare delivery for underserved communities and populations in remote areas. This paper proposes a routing strategy that incorporates synchronization constraints to increase the efficiency of MHC routing by utilizing a high-capacity resupply vehicle. Seven meta-heuristic methods (ALNS, Simulated Annealing, Ant Colony Optimization, Particle Swarm Optimization, Genetic Algorithm, Tabu Search) were developed to solve this complex combinatorial problem and a comparative study of them was conducted. The results obtained show that the synchronized routing model is more efficient in routing the MHCs and the comparative analysis serves as a framework for obtaining good solutions in shorter time periods. Analysis of Meta-Heuristics
15 – Network-theory based Approach to Assessment of Bidding Competition in Design Bid Build Highway Projects
Muhammad Ali Moriyani (UNC Charlotte)
Design-bid-build (DBB) is the predominant method of public highway procurement due to its potential to minimize costs, optimize project outcomes, and maintain procedural transparency. However, the intended benefits of DBB are contingent upon the adequacy of competition among bidders. Previous studies have indicated that higher or lower levels of competition can lead to unfavorable project outcomes, including monopoly or winner’s curse. Yet the primary indicator of competition employed by State Highway Agencies (SHAs) is the number of bidders, which overlooks the complex competitive dynamics, particularly the competitiveness of certain bidders. Recently, researchers have explored the potential of network theory to model competition among bidders. However, the application has been limited to collaboration networks rather than measuring competition at the contract level. This study addresses this gap by introducing a novel data-driven framework utilizing network theory to model competition that facilitates owners’ evaluation of competition adequacy at the contract level beyond the number of bidders. In the proposed approach, competition is modeled as a bidirected network of interconnected bidders, with edges representing their historical frequency of wins and losses. Various centrality measures in the proposed network provide distinct insights into bidder competitiveness. The proposed centrality measures are validated using Spearman’s rank correlation coefficient with bidding performance. The results indicate that these proposed measures exhibit stronger correlations with bidding performance than the number of bidders. This approach enables SHAs to obtain a more detailed assessment of contractor competitiveness and competition levels during project letting, thereby ensuring effective competitive bidding practices.
16 – Detecting Red-Flag Bidding Patterns in Low-Bid Procurement for Highway Projects with Pattern Mining
Muhammad Ali Moriyani (UNC Charlotte)
Collusive bid arrangement is among the most prevalent anti-competitive practices in design-bid-build projects, significantly impacting bid prices, which have largely remained undetected proactively by State highway agencies. The primary reason for the ineffectiveness of current practices and state-of-the-art methods in bid collusion detection is their substantial reliance on engineers’ estimates. There are various concerns regarding the use of engineers’ estimates, including inaccuracy and bid manipulative techniques employed by experienced collusive bidders, such as submitting bids with similar cost structures. This study proposes a novel data-driven collusion red-flag detection framework (CRFD) that utilizes pattern mining techniques and statistical tests to proactively identify contractors exhibiting red-flag patterns without relying on engineers’ estimates. The red-flag bidding pattern is defined as a significant difference in the winning rates of a contractor with and without the co-occurrence of other particular contractors. Additionally, a mechanism is proposed to incorporate potential influential factors into the CRFD to enhance detection power or examine possible collusion across different scenarios, such as spatiotemporal factors. The proposed method is anticipated to assist project owners in detecting early signs of bid collusion and ultimately aid in significantly improving their award decision-making processes.
18 – Mechanistic-Based Evaluation of Performance Thresholds for Balanced Mix Design Asphalt Surface Mixtures
Omar Othman (NC State University)
Balanced Mix Design (BMD) is an approach to design asphalt mixtures using performance tests seeking to balance the resistance to cracking and rutting. However, BMD is often decoupled from structural design considerations, leading to potential deficiencies in long-term pavement durability and increased costs due to premature failures. To address these issues, it is important to define BMD tests thresholds that are based upon performance under the intended conditions. The Virginia Department of Transportation (VDOT) has implemented BMD strategies for low- and moderate-traffic dense-graded asphalt mixtures. Their BMD specification relies on three empirical, yet practical tests: the Cantabro test, with a maximum mass loss of 7.5%, the Indirect Tensile Cracking test, with a minimum Cracking Tolerance Index (CTIndex) of 70, and the Asphalt Pavement Analyzer test, with a maximum rut depth of 8.0 mm. These thresholds were established through a benchmarking study completed in 2018 and are independent of the structure or intended traffic. The objective of this study is to refine the thresholds through the addition of fundamental testing paired with mechanistic performance predictions. For this effort, 18 Virginia-produced surface mixtures were evaluated using VDOT’s BMD tests as well as Dynamic Modulus (DM), Cyclic Fatigue (CF), and Stress-Sweep Rutting tests. Additionally, eight of these mixtures underwent DM and CF testing after long-term oven-aging, following NCHRP Project 09-54 protocols. The results helped calibrate the NCHRP Project 09-54 aging models for Virginia materials, which were incorporated into FlexPAVE™ simulations to establish traffic-based BMD thresholds aimed to enhance asphalt mixture durability and performance.
19 – Adversarial Attack towards Car-following Trajectory Prediction and Planning
Zijun He (NC State University)
Car following (CF) is a critical dynamics in traffic flow, which describes longitudinal movement of a vehicle stimulated by its preceding vehicle. To better understand CF dynamics, many CF models have been developed for traffic flow analysis and vehicle control. Motivated by the successful application of Artificial-Intelligence (AI) techniques across different fields, various AI-based CF models have been proposed to learn the CF behavior in the real traffic environment. Based on historical CF state, AI-based CF models can predict the future CF state of target vehicles. In a vehicle platoon mixed with autonomous vehicles (AVs), AVs will plan their trajectories based on historical and predicted movement of other vehicles to achieve driving efficiency and safety. However, AI-based CF models are vulnerable to adversarial attack. The attackers can apply carefully designed disturbance to malicious vehicles which might mislead the AI-based CF models to produce inaccurate predictions. Fed with such problematic prediction results, AVs may plan trajectories representing inefficient and unsafe maneuvers. In this work, we develop an attack framework targeting the AI-based CF models. The disturbance and its allocation will be carefully designed to optimize the attack outcome. We find that with the same attack budget, distributed attack can outperform centralized attack, i.e., allocating disturbance to multiple vehicles getting greater outcome.
20 – Optimizing the Mixture Design of Biochar-Fly Ash Concrete for Low-Carbon Construction
Jonathan Culpepper (Appalachian State University)
With global industrialization continuing to become more prevalent, the atmospheric CO2 content continues to increase. Concrete construction is a key part of development with cement production contributing 7% of the emissions related to the concrete industry. Studies have been done looking at using Supplementary Cementitious Materials (SCM) such as steel slag, silicon fume, and fly ash. Particle size, metal oxide content, and absorption capacity contribute to selection. Cement has long been supplemented with a type of SCM known as a Pozzolan, such as volcanic ash by the ancient romans. More attainable materials like fly ash produce a similar product and have been used as early as the 1930s. Class F fly ash obtained from a local source will be one of the SCMs used in this study. Other materials such as biochar, a carbon rich material derived from biomass undergoing pyrolysis with carbon sequestering properties, has been investigated as an SCM when certain biomasses are used. Arundo donax grass is the biomass of choice for this study due to its high regeneration rate, local availability, and the opportunity to repurpose a plant species with potential to harm other species. Additionally, the biochar used was produced by other graduate students at Appalachian State University. This study aims to investigate and determine the optimal cement replacement ratio of Class F fly ash and Arundo donax biochar by performing various tests (compressive strength, flexural strength, workability, slump, setting time, and flexural strength).
21 – Q-Loc: Visual Cue-Based Ground Vehicle Localization Using Long Short-Term Memory
Cole Malinchock (NC State University)
Q-Loc: Visual Cue-Based Ground Vehicle Localization Using Long Short-Term Memory, is a proposed method of utilizing pretrained neural networks to predict the location of an autonomous vehicle. It is a rapid and cost-effective localization method using a sensor ubiquitous in autonomous systems: cameras. We introduce a system that uses vision-based machine learning techniques to detect common landmarks in camera streams and subsequently predict location. The system employs a Faster-RCNN object detection model for landmark identification and a custom Long Short-Term Memory neural network architecture for vehicle localization based on the bounding boxes of the detected landmarks. A Kalman Filter, commonly used in localization, is incorporated to enhance prediction accuracy. To assess Q-Loc’s feasibility and effectiveness, we implemented a prototype on a small-scale autonomous vehicle platform equipped with an NVIDIA Jetson Orin and a Zed2i camera, conducting trials on North Carolina State University’s Centennial Campus. The campus’s complex and varied landscape allows us to evaluate the system’s robustness, accuracy, and real-world application potential. Across the 750 meter path, the prototype’s results reveal that Q-Loc achieves a mean localization error of 16.57 meters, with a standard deviation of 18.79 meters, demonstrating consistent accuracy across tests. The localization error ranges from as low as 0.11 meters, showing the method’s high precision in optimal conditions with low tree cover and more than one landmark identifiable, to a maximum error of 102.49 meters under challenging scenarios with little to no landmarks detected and high tree coverage, highlighting areas for future refinement.
22 – Public Charging Infrastructure for Power Mobility Devices to Enhance Mobility and Inclusion
shokoufeh Valadkhani (NC State University)
In the U.S., 12.1% of the population experiences a mobility disability, with Power Mobility Device (PMD) users traveling 73% less than an average American walks, highlighting significant mobility challenges and barriers to social inclusion. Developing public charging infrastructure for PMDs is essential to enhance mobility and promote community engagement. The researchers at NCSU developed the charging infrastructure for PMD units consisting of an IoT-integrated universal charger for PMD batteries, a connected battery- and PMD-data acquisition and monitoring system, and a mobile app with AI-driven energy consumption estimators. This integrated system aims to empower PMD users, improving their daily mobility, social interaction, and community engagement. Intended for both 120-V and 220-V grids, this charger features a lightweight design, high efficiency, a programmable output range of 50–330W, and robust safety characteristics. An optional Data Acquisition and Sensing System (DASS) monitors critical parameters, including battery voltage, current, and temperature, as well as GPS-based location and environmental parameters, which can be uploaded to a dedicated database for customizing AI-based energy estimators. The mobile app assists wheelchair users by locating accessible public charging stations, planning optimal routes, displaying real-time battery levels, and monitoring the charging process. The app provides real-time battery status and charging cycles when connected to DASS. The App route planner estimates distance, travel time, and battery energy needed based on user-specific data via DASS, enhancing accuracy compared to a generic estimator. The app adheres to the strict recommendations of the Web Content Accessibility Guideline (WCAG) 2.1 AA standard for accessible design.
23 – Pumped Concrete Point of Placement Air System Evaluation
Sunday Akanji (UNC Charlotte)
Specifications regarding the point at which concrete is sampled and tested for air content vary by agency. Some agencies, including the North Carolina Department of Transportation (NCDOT) require point-of-placement testing for acceptance, with the concrete sampled and tested after pumping. Recent research has indicated that although a drop in air content is often measured after pumping, the air bubbles pressurized into solution during pumping can return minutes or hours after pumping. This phenomenon is documented in Ley et al. of Oklahoma State University in several publications. Laboratory and field studies on the air system behavior before and after pumping have resulted in a number of agencies changing their specified point of air content testing from the point of placement (which interrupts work and presents safety and logistics challenges) to testing at the truck before placement. NCDOT is interested in exploring modifications to their specifications related to testing pumped concrete. This work aims to investigate typical NCDOT AA (structural) concrete mixtures with different local materials, pumped using several boom configurations, to assist NCDOT in determining whether they desire to modify their specifications regarding air content testing.
24 – Utilizing Artificial Intelligence for Traffic Sign Detection and Classification for Safe Operation of Connected and Automated Vehicles in Rural Areas
Mohammed Zakaria (NC A&T University)
This research presents an approach to traffic sign detection and classification tailored for autonomous driving in rural environments. Utilizing the state-of-the-art deep learning techniques, we developed a model capable of identifying and categorizing traffic signs into three primary classes: regulatory, warning, and guide signs. Our study addresses the unique challenges posed by rural roadways, where traffic sign detection is crucial for safe autonomous vehicle operation. To ensure relevance and accuracy, we curated a diverse dataset by collecting images from Google Maps Street View, focusing on rural settings. This dataset was meticulously annotated to train our AI model. The developed model was chosen for its superior performance in real-time object detection, a critical factor for autonomous driving applications. Our model’s streamlined classification into three broad categories allows for efficient processing while still providing essential information for navigation and safety decisions. This research contributes to the growing body of work on computer vision applications in autonomous driving, with a specific focus on addressing the needs of rural transportation infrastructure. Our findings have implications for improving the safety and reliability of autonomous vehicles operating outside of urban centers, potentially bridging the gap in autonomous driving capabilities between urban and rural environments.
25 – Using Ground Penetrating Radar to monitor the condition of Pavement Structure Sublayers
Ngonsah Obasi Ndikum Sally (UNC Charlotte)
Moisture content and density are crucial parameters for controlling and assessing the quality of pavement structures, particularly the sub layers such as the base, subbase, and subgrade. Ground Penetrating Radar (GPR), a non-destructive methodology, was used to scan two flexible pavement sites in North Carolina to monitor and determine the quality of the pavement structure layers and the top 2.44 m (8 ft) of the embankment at these sites. The frequencies and amplitudes generated from the scans were used to predict the dielectric constant of each layer material, from which the thickness of each layer within the pavement structure was estimated. Preliminary evidence indicates that the estimated thicknesses from the GPR results match the design thicknesses of the asphalt concrete layer of the flexible pavement at the sites. The ongoing study involves using the predicted dielectric constants to estimate moisture contents and densities of the subbase and subgrade layers, as well as the embankment at different depths, to assess the current integrity of subsurface below the asphalt and base layers of the pavement structures. The outcome of this non-destructive and non-intrusive monitoring methodology can be leveraged to improve pavement construction and maintenance strategies.
26 – Long term Performance of MSE Wall used as Bridge Foundation
Adams Familusi (UNC Charlotte)
A 7m (23 ft) high and 9m (30ft) wide instrumented mechanically stabilized earth (MSE) retaining Wall was constructed to support a bridge foundation as an alternative to pile foundation due to site configuration restrictions. Pressure and settlement data were collected from the sensors installed below the MSE wall and the bridge foundation for approximately six (6) months, starting from the construction period. Numerical analysis of the of the wall’s performance using FLAC-3D, a finite difference computer program, was conducted and compared with pressure and settlement readings from the embedded sensors. The simulated pressure and settlement values from the numerical analysis were about 15% and 3% less than the measured pressure and settlement from the sensors, respectively. It was observed that in addition to the interface cohesion and angle of internal friction, one of the main factors contributing to the pressure distribution and settlement around the MSE wall is the value used in the simulation for coupling normal and shear stiffness between the selected fill material and MSE wall facing. This is largely dependent on the method of installation and type of MSE wall facing. Parametric studies are ongoing to better understand the effect of the different reinforcement types on the behavior of the MSE wall and to inform the longer-term performance of the MSE wall towards developing a guide for future reference.
27 – What’s the Fuss About the Bus?: How Parent Perceptions of Transit Affect Youth Transit Use in Chapel Hill, North Carolina
Rachel Oommen (UNC Chapel Hill)
The Chapel Hill-Carrboro area of North Carolina benefits from a unique transportation dynamic wherein a 24-route bus system (Chapel Hill Transit), funded in large part by the local university, provides service to both university students and the general public, completely free of charge. Although the combined population of the two towns is under 100,000 residents, the area’s youth have the type of mobility typically offered to those of larger cities. Even so, the design, structure, and culture of the area lends itself to automobile dominance. In light of these conflicting realities, understanding how individuals relate to transportation can highlight avenues for intervention to encourage greater transit use. Thus, my research question is: What are the factors impacting youth ridership of transit in the Chapel Hill-Carrboro area? I hypothesize that Chapel Hill-Carrboro parents have pro-transit ideologies, but in practice caution their adolescent children against bus ridership, resulting in low ridership among this age cohort. It is so rare to grow up in a town that provides free public transit. Youth that grow up in this context are uniquely situated to continue a life-long connection to transit, as the cost barrier is removed for themselves and their peers. Thus, learning the reasons why adolescents do or do not take advantage of such a resource will be crucial for Chapel Hill Transit in understanding not just what they’re doing well to retain youth riders, but also what changes could be made to support a transit lifestyle for them in adolescence and beyond.
28 – Efficient Traffic Monitoring Using High-Resolution Aerial Images
Tewodros Gebre and Leila Hashemi Beni (NC A&T University
Remote sensing technology has become increasingly important for real-time, effective monitoring approaches in transportation management. One of the prominent methods of remote data acquisition involves the utilization of drones, which provide high-resolution aerial imagery to enhance data collection and analysis.. However, the processing of high-resolution images, a crucial component of remote sensing, poses significant computational challenges. The sheer size of these images, combined with the intense computational demands of object detection algorithms, can result in prolonged processing times and substantial computational costs, hindering the effectiveness of remote sensing in transportation applications. This study aims at processing high-resolution aerial images for traffic monitoring. We have developed an Adaptive Input Sampler (AIS) to optimize input data volume while maintaining detection performance in remote traffic sensing applications. The AIS utilizes pixel change monitoring and predictive algorithms based on historical object locations and speeds, enabling historical success-based object trajectory extrapolation. By detecting vehicles and selectively sampling image areas, the AIS reduces data input for downstream object detection systems, reducing computational load and improving inference speed. Our experiments demonstrate that the AIS achieves a 96.40% traffic counting accuracy and 95.60% classification accuracy while reducing data size for analysis by up to 79.00%. The AIS presents a promising solution for managing large datasets in traffic monitoring applications, enhancing computational efficiency, and speeding up inference results.
29 – Graph-Powered Defense: Controller Area Network Intrusion Detection for Unmanned Aerial Vehicles
Reek Majumder (Clemson University)
In the last decade, Unmanned Aerial Vehicles (UAVs) have shown significant growth in the network of services, including product delivery, agriculture, environment monitoring and many more. However, UAVs are not secure enough against cyber-attacks, especially on the Controller Area Network (CAN) bus. The CAN bus is a general-purpose vehicle-bus standard designed to allow microcontrollers and computers in vehicles to communicate with each other, primarily connecting different Electronic Control Units (ECUs). In this study, we focus on solving some of the most critical security weaknesses in UAVs by developing a novel graph-based intrusion detection system (IDS) leveraging the Uncomplicated Application-level Vehicular Communication and Networking (UAVCAN) protocol. First, we decode CAN messages based on UAVCAN protocol specification; second, we present a comprehensive method of transforming tabular UAVCAN messages into graph structures. Lastly, we apply various graph- based machine learning models for detecting cyber-attacks on the CAN bus, including graph convolutional neural networks (GCNNs), graph attention networks (GATs), Graph Sample and Aggregate Networks (GraphSAGE), and graph structure-based transformers. Our findings show that inductive models such as GATs, GraphSAGE, and graph-based transformers can achieve competitive and even better accuracy than transductive models like GCNNs in detecting various types of intrusions, with minimum information on protocol specification, thus providing a generic robust solution for CAN bus security for the UAVs.
30 – Real-Time Animal Detection System to Enhance Automated Driving Safety in Rural Roads
Sita Ram Prasad Martha (NC A&T University)
Animal-vehicle collisions are a significant safety concern particularly in rural communities, resulting in numerous fatalities, injuries, and economic losses annually. Traditional methods like static animal crossing signs depend heavily on driver awareness, making them insufficient for preventing sudden encounters with wildlife. This research presents a real-time animal detection system that can be used for enhancing the safety of automated driving or advanced driver-assistance systems. By leveraging deep learning models, the developed system can accurately detect high-risk species, such as deer and moose, and provide timely alerts to avoid collisions. The system was trained on a comprehensive dataset that includes images captured in varied lighting and environmental conditions, ensuring accurate detection in low visibility settings typical of dawn, dusk, and nighttime driving. This ongoing research seeks to demonstrate high accuracy and responsiveness, with the potential to significantly improve road safety.
31 – Accessing Current and future Implementations of Transit-Oriented Development related Social Housing in the United States.
William Evans and Rongfang Liu (NC A&T University)
This research will explore how integrating social housing policies with Transit-Oriented Development (TOD) can reshape housing affordability in the United States. By examining affordable housing policies across three areas of the United States—Cleveland, OH, Charlotte, NC, and the River COG in Connecticut —this study aims to reveal the potential of U.S. housing policies to support social housing near transit hubs. Through these case studies, the research seeks to illuminate pathways for transforming housing policy to support accessible, affordable housing solutions tied to robust public transit, ultimately offering insights to shape more inclusive urban development strategies.
32 – Teaching Safety: Analyzing North Carolina’s Driver Education Program and its Approach to Young Drivers’ Perceptions and Interactions with Vulnerable Road Users
Annalisa Sims (UNC Chapel Hill)
My project will analyze the North Carolina Driver Education program and assess how young drivers are taught to interact with Vulnerable Road Users (VRUs). It will evaluate not only the more technical skills of driving in a multi-modal environment but will also assess the way the current curriculum and training depicts vulnerable road users, thereby shaping perceptions of drivers. I will look at NC’s written curriculum and compare it to other states’ that have been identified as exemplary in their inclusion of interactions with VRUs. I will also interview driver education instructors to better understand how they deliver the training, any gaps they see in the current curriculum, and their own perceptions of VRUs. The final deliverable of this project will be a policy paper that provides recommendations for the driver education program. This project aims to support NCDOT’s 2024 Strategic Highway Safety Plan, Emphasis area: “Develop and promote public education programs that support education for all road users on safety for pedestrians, bicyclists, and personal mobility users. Strategy: Incorporate interactions with pedestrians, bicyclists, and users of personal mobility in the driver education curriculum.”
33 – Utilizing Artificial Intelligence Tools to Assess Physical and Digital Infrastructure for Safe Connected and Automated Vehicle Operations in Rural Areas
Mohammed Zakaria (NC A&T University)
The safe operation of connected and automated vehicles (CAVs) in rural areas presents unique challenges due to the distinct characteristics of rural roadway infrastructure. This research focuses on assessing both the physical and digital infrastructure components crucial for CAV deployment in rural settings. Our study evaluates key physical infrastructure elements, including road surface conditions, lane markings, traffic signs, and traffic lights. We employ advanced deep learning models to analyze and assess the quality and readiness of these components for CAV operations. On the digital infrastructure front, we examine critical elements such as network connectivity, GPS accuracy, high-definition map availability, and the presence of roadside sensors. These digital components are essential for enabling reliable communication, precise localization, and real-time data exchange for CAVs in rural environments. By combining assessments of both physical and digital infrastructure, our research provides a holistic view of the current state of rural roadways for CAV deployment. This comprehensive evaluation helps identify gaps, challenges, and opportunities for improving rural infrastructure to support the safe and efficient operation of CAVs. Our findings contribute to the understanding of rural-specific needs for CAV infrastructure and offer insights for policymakers, transportation agencies, and technology developers. This research aims to bridge the urban-rural divide in CAV readiness and promote equitable access to advanced transportation technologies across diverse geographic areas.
34 – Mobilizing Mutual Aid: A toolkit for community resource exchange to minimize travel needs
Audrey Compiano (UNC Chapel Hill)
The 2022 National Household Travel Survey indicates that people travel beyond a typical walkshed to access resources daily. The necessity of transportation for accessing resources poses an issue for people experiencing transportation insecurity, which, disproportionately, is people experiencing poverty. Affected households’ ability to access resources can be further exacerbated by time poverty, affordability of resources, and relational impacts such as feeling guilty about relying on others for transportation. The inadequacies of current transportation systems warrant the need for alternative solutions that minimize travel needs and address the implications of poverty. Resource exchange through mutual aid projects is a way to leverage existing community resources, minimize travel, eliminate costs, and build solidarity over shared struggles. Mutual aid is an organizational model where collectives coordinate non quid pro quo exchanges of resources at no cost to members, fostering community agency and resilience. Existing discourse does not position resource exchange mutual aid as a transportation insecurity solution, resulting in a lack of supportive resources to implement mutual aid projects that facilitate exchange while minimizing the need for travel. This research will focus on the question: What tools and strategies can be employed to develop resource exchange mutual aid projects that minimize the need for travel in North Carolinian communities? A thorough review of mutual aid projects will be conducted through case study review and interviews, with a focus on tools and strategies for creation and implementation. Research will culminate into a toolkit for community members that supports the replication of mutual aid projects.
35 – Assessing Arterial Performance Using Probe Data: A Comparative Analysis of Capital Boulevard and Concord, NC
Sk Nahia Ahsan (NC State University)
With the increasing availability of probe data, there is a significant opportunity to enhance our understanding of arterial performance. Probe data provides detailed insights into travel rates, which are crucial for assessing arterial performance and the impacts of signal control. In this study, we are analyzing one month of probe data for Capital Boulevard in Raleigh, NC, in conjunction with Automated Traffic Signal Performance Measures (ATSPM) to see how much better we can understand the system’s performance. We want to see whether the findings from this new assessment align with those from our previous study of an arterial in Concord, NC. We again see evidence that the 5th percentile of travel rate can serve as a useful indicator of arterial performance. This result is attributed to the behavior of faster-moving vehicles, whose travel times are more immediately affected by increasing traffic density. By incorporating cumulative distribution functions (CDFs) of travel rates—particularly the 5th, 50th, and 95th percentiles—both analytical and simulation-based models can more accurately represent network performance. Our analysis suggests that CDFs offer a more comprehensive depiction of traffic conditions compared to traditional delay metrics at individual intersections. In this case, we are using TransModeler to develop a simulation model of the arterial. Moreover, in this instance, we are seeking to use machine learning to identify ways in which system performance can be enhanced by making probe-driven enhancements to the way in which the traffic signal control system responds to the temporally- and spatially-varying demands.
36 – Preliminary Findings on RP2023-02 Balanced Mix Design for North Carolina Project
Pablo Vestena (NC State University)
Since implementation of Superpave Mix Design, states have moved towards an asphalt mixture design approach explicitly concerned with preventing distresses and balancing performance. Throughout the years, some agencies have added performance testing to its protocols, either at design, assurance or quality check phases. In the current specification, NCDOT has only limited rutting evaluation for designing mixtures. To feasibly add new testing protocols, and further recommend thresholds, it is paramount that the current performance properties of North Carolina mixes is well understood and that the State understand the approach that contractors take to mixture design. This work aims to benchmark 14 North Carolina surface mixes (RS9.5B, RS9.5C, and RS9.5D) in terms of simple and inexpensive Balanced Mix Design (BMD) testing (IDEAL-CT and APA), and fundamental tests. Besides that, a survey was conducted to identify the status of North Carolina asphalt plant contractors in terms of size, operation location, training of personnel, and familiarity with BMD protocols. The cracking results suggest that there are no differences between RS9.5B and RS9.5C mixes; however RS9.5D presented unexpected worse performance for the selected testing protocols. In terms of rutting, the actual thresholds are well above most results, showing that there is room to improve the cracking side without hurting the overall performance. Ultimately, with the understanding of the collective results, the research team identified possible thresholds for design of North Carolina asphalt mixes (laboratory-mixed laboratory compacted) and identified design protocols that could enhance cracking with minor impact on rutting, as COAC and AAMD.
37 – Characterizing the Unique Properties of Natural Fiber Composites Nondestructively
David Warren (Elizabeth City State)
Aircraft manufacturers need sustainably derived thermoplastic composites in order to build environmentally friendly aircraft so that the environmental impacts of air commercial transport is reduced. This research develops non-destructive test methods and structural health monitoring approaches for these materials, aiming to determine what nondestructive evaluation techniques are most suitable for characterizing these natural fiber composites. Considering the non-conductive and dielectric properties of these natural fiber composites, identifying effective NDE methods can pose a challenge. This study investigates these composites using Terahertz Time Domain Spectroscopy, Laser Doppler Vibrometry, an LCR Meter, and ultrasonic techniques to characterize acoustic and electric properties of the Flax Fiber composites. The research also involves optimizing the scanning equipment’s parameters in order to achieve the ideal setting for repeatability of data collection. Equipment and software include MATLAB, LabVIEW, SolidWorks, Ultrasonic Transducers, Pulser Receiver, Terahertz systems, Laser Doppler Vibrometer, LCR Meter, and 3D Printing. A Terahertz Time Domain Moisture Experiment identified that Terahertz scanning can effectively detect moisture content in natural fiber composites. A Terahertz distance offset experiment identified the optimum scanning distance a material should be analyzed away from the lens regarding maximum amplitude intensity. A laser doppler vibrometry experiment identifies how guided waves propagate and interact with the natural fiber composite using non-contact vibrational methods. An impedance, inductance, and capacitance test on a wooden tongue depressor identifies the harmonic resonance, dielectric properties, and electrical properties of natural wooden fibers. These investigations successfully identified effective nondestructive evaluation methods, and characterized the unique properties of natural composites.
38 – Analyzing driving behavior distraction during animal crossing in rural road
Amir Milad Javadi (NC A&T University)
The rapid advancement of autonomous driving technology provides a potential solution to improve transportation in rural areas. The interaction between the driver and the autonomous vehicle has an impact on road safety. Rural roads have their unique characteristics (i.e., narrow roads, steep hills) and conditions (i.e., animal crossing). This research aims to use the cutting-edge eye tracking technology integrated with the vehicle in the loop, human in the loop driving simulator to investigate the driver’s behavior in rural road driving. Findings of the research will help improve autonomous vehicle design and improve rural road safety.
39 – 3D Printed VTOL Mini Flying Wing
Rylan Penley (Elizabeth City State)
The overall objective of this project is for a proof-of-concept vertical take-off and landing flying wing with theoretically better efficiency than a typical fixed wing UAV, as well as having the landing and transportation capabilities of a quadcopter UAV while being faster. The success of this objective would help to ensure a pathway for future UAVs that can be utilized for a multitude of tasks and services. Some of these possible tasks could include, grocery delivery, emergency delivery, search and rescue, and observations. A UAV with combined characteristics of a quadcopter and a fixed wing while also being lighter and quicker could become much more successful than common UAVs at those previously stated tasks. All of these would further compliment the ease of manufacturing, as well as the fact that the body of the UAV would be made entirely from 3D printed materials, therefore potentially cutting down on the overall costs of drone manufacturing.
40 – A Probabilistic Evaluation of Recycled Binder Replacement Limits
Wei Xie (NC State University)
Reclaimed asphalt pavement (RAP) is widely used in US pavement construction for its economic and environmental benefits, but the oxidized binder in RAP can affect pavement performance. While state agencies typically limit recycled binder replacement (RBR) percentages, previous studies have used deterministic analyses that don’t fully account for variability in virgin and RAP binder properties. This study conducts a probabilistic evaluation of RBR limits for North Carolina asphalt mixtures, where current specifications allow up to 0.4 RBR with different virgin binder grade requirements based on RBR level. The research analyzed NCDOT Quality Assurance data from 361 virgin binder samples (PG 64-22 and PG 58-28) collected between 2022-2024, along with 33 RAP binders extracted from various North Carolina stockpiles. Bootstrap resampling was employed to expand both virgin and RAP binder datasets to 10,000 points each, enabling comprehensive analysis of blended binder properties using linear blending charts. Complementary testing of a subset of blends of virgin and RAP binder was conducted to verify the accuracy of the linear blending charts for NC materials. The study assessed the reliability of meeting PG 64-22 grade requirements at various RBR levels and virgin binder grades, while also evaluating the statistical similarity between PG 64-22 virgin binders and blends of PG 58-28 and RAP binder at different RBR values to gain further insight. This analysis, combined with low-temperature grading results, aims to develop robust RBR specification recommendations for North Carolina.
41 – Secure Navigation in Connected and Automated Urban Transportation
Ahmad Mohammadi (NC A&T University)
GPS spoofing attacks pose significant threats to the safety and security of Connected and Automated Vehicles (CAV), as it can manipulate navigational data and mislead vehicles into erroneous paths, potentially leading to dangerous situations. To address this problem, we developed a detection algorithm that utilizes onboard sensor data from vehicle to detect these threats in real-time and more effectively. The proposed algorithm processes data from the speedometer and inertial measurement unit (IMU) using artificial intelligence (AI) to forecast vehicle movements. These forecasts are then compared against GPS data to detect various spoofing scenarios, including turn-by-turn, stop, and overshoot attacks. Moreover, the algorithm is equipped to identify sequences of multiple biased attacks that, although individually undetectable, cumulatively lead to significant deviations in vehicle rout over time and lead to dangerous situations such as accidents caused by driving in wrong direction and kidnapping by going to a wrong destination. To assess the accuracy of this algorithm, we simulated four distinct spoofing scenarios—turn-by-turn, overshoot, stop, and multiple biased—from real-world data sourced from the Honda Research Institute Driving Dataset (HDD). Multiple tastings were done using various driving conditions such as days and nights, different speeds in both urban and rural areas. The data were then analyzed by the algorithm. This testing confirms that our model effectively identifies these spoofing attacks with high precision: it detected turn-by-turn, stop, overshoot, and multiple biased attacks with accuracies of 100%, 99.95%, 99.91%, and 97.83%, respectively.
42 – Investigating Automated Shuttle Readiness for Rural Areas: A North Carolina Case Study
Oladimeji Alaka and Dr. Venktesh Pandey (NC A&T University)
Recent decades have seen the potential of automated shuttles in addressing transportation needs for vulnerable communities; however, the infrastructure and community readiness in urban and rural underserved communities for the deployment of these shuttles remains unexplored. This research evaluates the level of readiness of rural counties in North Carolina for implementing autonomous vehicle (AV) technology, focusing on physical infrastructure, digital infrastructure, and social vulnerability using a range of indicators derived from secondary data sources, including road quality, bridge conditions, broadband availability, and socio-economic factors. The geospatial analysis reveals a diverse landscape of readiness across the counties, with variations in physical and digital infrastructure and social vulnerability indices. Despite being rural and located in coastal regions, Camden County emerged as a leading candidate for AV deployment due to the better availability of the internet, good road conditions, and a population in need of autonomous shuttles. This was followed by Halifax, Chatham, and Bladen counties. To further understand regional differences, we used clustering analysis to identify distinct clusters within census tracts that reflect varying readiness levels. While some clusters are well-positioned for early AV deployment, others require infrastructural improvement. Conversely, counties with higher social vulnerabilities indicate a potential need for autonomous shuttle interventions to enhance the livelihood of the population in need of these services. The study findings emphasize the importance of a multi-weighted analysis for readiness assessments that can guide investments and strategic interventions and the potential of data-driven approaches that can be generalized to other states for determining readiness.
43 – What do Applicants Really Need for the Grant Application Process?
Brooke Kongmany (UNC Chapel Hill)
What do grant applicants really need for federal grant applications? Increasing professionalization within the transportation space has increased the complexity of USDOT discretionary grant applications. The application process itself is very complicated with specific details and requirements that applicants must adjust to in order to get funding. Before being considered for funding, potential grantees must submit several documents detailing every component of their proposed project and must go through eligibility requirements before it is passed along for evaluation. All of this complexity can hinder many applicants from obtaining funding for their projects and forces them to redo and reapply for these grants during the next grant cycle. This project will analyze and examine what applicants really need for the grant process and can inform ways to simplify the process in order to relieve some of the burden placed on applicants. This analysis will delve into discretionary grant programs from the FHWA, FRA, and FTA and will include a cross analysis of their similarities and differences along with pros and cons of application materials for both the Federal and applicant sides to conclude what is really necessary and helpful for a successful grant application.
44 – Trajectory Prediction under GPS Spoofing Attacks for HATS: Leveraging Synthetic Data for Enhanced Resilience
Frederick Owusu-Ambrose (NC A&T University)
Highly Automated Transportation Systems (HATS) have gained attention and adoption in the transportation sector as they improve safety by reducing total reliance on human drivers. However, the inherent risk of the capabilities that enable these automations provides avenues for malicious attacks, which can cause HATS to deviate from their intended routes. In this research, we develop an advanced predictive modeling framework designed to forecast the future states of the HATS under adversarial conditions. We predict plausible vehicle trajectories post-attack by leveraging machine learning, state estimation methods, and time series analysis. Using synthetic data to simulate GPS spoofing scenarios, we create a controlled environment to observe system vulnerabilities, analyze potential attack patterns, and forecast likely outcomes. This approach facilitates understanding of disruption impacts, enabling efficient deployment of first responders and informing preemptive adjustments to mitigate safety risks. Our work underscores the importance of traffic safety and system integrity in urban environments, highlighting how accurate forecasting of vehicle trajectories post-attack can significantly enhance the resilience of HATS. This capability strengthens system reliability, contributing to public trust by demonstrating the system’s ability to anticipate and respond to unexpected disruptions. This research thus supports a foundation of trust, ensuring that autonomous vehicles remain dependable and resilient components of modern transportation networks, even in the face of cybersecurity threats.
45 – Evaluating the applicability of UAV-based sonar for bridge substructure monitoring.
Quinn Keefer (Appalachian State University)
Currently, NCDOT manages approximately 13,700 bridges, with 8.4 percent of these bridges considered in poor condition. Despite systematic inspection protocols, traditional methods have limitations, such as insufficient monitoring of upstream scour features. UAV based sonar offers considerable benefits compared to traditional methods. The current inspection protocols involve taking soundings at specific points around bridge substructures and making additional measurements after high water events to track changes in the streambed profile. UAV sonar systems are able to capture thousands of points rapidly, yielding a much more detailed view of the streambed and bridge substructure. The Appalachian State Drone team conducted a case study on Huffman bridge along Rhodhiss Lake using a DJI Matrice 600 Pro equipped with an ECT 400S echosounder. This data was validated with multiple ground control measurements taken from a canoe using a measuring rod and D-GPS. Results from the survey were extremely encouraging, with the R-squared of the ground truth and UAV measurements being 0.9. Following the initial data collection, Hurricane Helene swept through Western North Carolina leaving massive damage. To further test the UAV sonar system, the team returned to Huffman bridge and conducted a second data collection. Preliminary results showed massive changes in the bathymetry of Rhodhiss lake, demonstrating a practical application of UAV based bathymetry. Capturing similarly detailed data using traditional methods would require substantially more labor to collect.
46 – Wireless Jamming Mitigation Methods
Bryce Herring (Fayetteville State University)
Wireless jamming attacks pose a threat to the integrity, reliability, and security of modern wireless communication systems, impacting military infrastructure, emergency services, and public safety networks. These attacks interfere with signal transmissions, which can not only compromise critical and highly sensitive data, but also endanger the lives of citizens should there be a significant delay in emergency services. This research project aims to explore effective methods for anti-jamming attacks, along with ensuring wireless communication remains constant and functional under the most hostile environments. To study these effects, we are utilizing HackRF, a software-defined radio, to simulate and analyze various jamming scenarios across different frequency bands. HackRF enables fine control over signal manipulation, allowing for real-time parameter adjustments, filtering, and decimation, which support high-fidelity data collection and analysis. Key methods include FM demodulation and Python-based signal processing to isolate and assess interference impacts on essential communication signals. Our research aims to create adaptive and responsive strategies that reinforce wireless network resilience, such as frequency hopping and power modulation techniques. In summation, we plan to use HackRF as the first stepping stone towards identifying and mitigating the impact of a wireless jamming attacks through the rigorous testing of data and constant analysis of radio signals. Findings from this project will contribute to the growing field of cyber resilience, offering insights into practical mitigation measures against wireless jamming threats.
47 – Urban Air Mobility Flight Operations Risk Assessment Based on Battery Energy Constraint
Yonas Ayalew, Abdul-Rauf Nuhu, Lydia Zeleke, Vahid Hemmati, Abdollah Homaifar, and Edward Tunstel (NC A&T University)
As cities become increasingly congested and environmentally conscious, the need for innovative solutions to address transportation challenges has never been more pressing. Urban Air Mobility (UAM) envisions a future where electric Vertical Takeoff and Landing (eVTOL) aircraft, and other aerial vehicles seamlessly integrate into existing transportation networks, offering efficient, safe, and sustainable alternatives to traditional modes. In this research study, risk assessment of UAM operations and routes considering separation automation and mission structure sensitivity based on energy demand is presented. Identified UAM potential routes in major US metropolitan areas are simulated using agent-based simulation considering level flight conflict management. We evaluated the energy consumption of a hexacopter eVTOL vehicle for all the routes with a mission parameter sensitivity study for operational flexibility and assessed the associated risk level of the vehicle’s overall flight energy consumption using statistical metrics. Our results demonstrate that the feasibility and energy demand of the flights can be affected greatly by the vehicle, range of operational routes, conflicts, and different parameter values of the mission structure. This study highlights that a mission parameter-based sensitivity study, that respects the minimum operational and performance regulations, can provide information about possible safety degradation in response to the choice of different airspace structures. Additionally, the statistical approaches reveal the level of risk associated with high energy-demanding flights for the potential routes in two metropolitan regions. These findings provide air transportation modelers with crucial insights for enhancing strategies aimed at accelerating the implementation of advanced mobility and mitigating their operational hazards.
48 – Security Risks in Urban Air Mobility: Evaluating Trojan Attacks on Autonomous Landing Systems
Reza Ahmari (NC A&T University)
In this study, we explored the vulnerabilities of autonomous landing systems in Urban Air Mobility (UAM) vehicles, specifically examining the risks posed by Trojan attacks on deep learning models used for navigation. Trojan attacks are a significant cybersecurity concern, as it inserts hidden triggers within Convolutional Neural Networks (CNNs), leading to misclassifications under specific conditions while maintaining normal functionality under typical operations. We focused on assessing the susceptibility of Urban Autonomous Aerial Vehicles (UAAVs) to these attacks, using models like DroNet, which are widely employed for real-time obstacle avoidance. To investigate this, we collected a custom dataset of over 5,000 images of landing pads, simulating both clean and Trojan-triggered conditions. Our deep learning model was trained on this dataset, and its performance was evaluated under both normal and triggered scenarios. The results revealed a substantial drop in accuracy, from 96.4% on clean data to 73.3% on Trojan-infected data, illustrating the severe impact of Trojan attacks on model reliability, particularly during critical phases like landing. These findings highlight the pressing need for robust defense mechanisms to mitigate Trojan-induced vulnerabilities and stress the importance of further research into enhancing the security of autonomous systems in UAM applications. These applications include navigation during takeoff and landing, obstacle avoidance, and communication with ground control. UAM vehicles have the potential to revolutionize passenger transport, emergency services, cargo delivery, and surveillance. Ensuring the security of these systems is essential, as any vulnerability could endanger lives, sensitive data, or critical infrastructure.
49 – Spatial Patterns in Logistics Warehouse Development in North Carolina
Graham Bishai (UNC Chapel Hill)
The rise in online shopping has driven construction of fulfillment and distribution centers that can process high volumes of freight headed for doorsteps each day. We analyze the location of these facilities in North Carolina and document a trend towards logistics sprawl, with some countervailing trends toward proximity logistics in large population centers, to facilitate last-mile delivery. We compare the North Carolina results with studies of other North American regions. We couple the geospatial data analysis with interviews with land use planners to understand key factors in the development approval and siting processes. We find that in North Carolina, most logistics facilities are built on land already zoned for industrial activities and therefore are developed by-right. Because of this, local communities and planners have limited mechanisms to mitigate the traffic and road safety impacts of proposed logistics developments. Interviews found varied local attitudes toward logistics facilities across jurisdictions. In some localities, local leaders entice logistics development as part of their economic development strategy, in others such development is viewed more skeptically. As warehouses increasingly develop in spatially deconcentrated and unplanned patterns, we found only limited regional coordination on freight planning to be occurring between North Carolina counties and municipalities.
50 – Enhancing Corrosion Resistance in Civil Infrastructure: The Role of Zirconium-Doped Blast Media in Protecting Transportation Assets
Ismail Megri (NC State University)
The application of zirconium-doped blast media presents a significant advancement in addressing corrosion problems within civil transportation infrastructure, such as bridges, highways, and tunnels. Corrosion poses a substantial threat to the structural integrity and longevity of these critical assets, often leading to costly repairs and safety hazards. By integrating zirconium-treated garnet media into the surface preparation process, this innovative approach not only enhances the adhesion of protective coatings but also actively delivers corrosion inhibitors during blasting. The study reveals that larger particle sizes in the blast media yield a remarkable 28% reduction in corrosion compared to untreated controls, highlighting the effectiveness of this method in real-world applications. This strategy not only improves the performance of protective coatings but also offers a proactive solution to mitigate the effects of environmental factors that contribute to corrosion. As civil transportation infrastructure continues to face challenges from moisture and corrosive agents, the adoption of zirconium-doped blast media could significantly extend the service life of these structures, ensuring safer and more reliable transportation systems for the future.
51 – Impact model validation and case study on overheight collisions with prestressed concrete girder bridges using finite elements.
Seth Cathey (UNC Charlotte)
The accidental collision of prestressed concrete (PC) girder bridges from overheight construction vehicles and objects such as dump trucks with raised beds or excavators on trailers presents engineers with the challenge of evaluating residual strength and serviceable life from field observations of visible damage. Accepting a damaged girder based on visual inspections exclusively may contribute additional safety concerns since damage mechanisms critical to ductile behavior in prestressed concrete, such as strand debondment or prestress loss, can be present without indicative visible indicators. One of the overarching goals of RP2023-06 aims to leverage practical tools and guidance for damage assessment on the strength and durability of laterally-impacted PC girder bridges from parametric finite element simulations and statistical methods using high-fidelity LS-DYNA models which are capable of predicting damage mechanisms (e.g., spalling, cracking, and inelastic deformation). This poster presents validation of the dump truck bed impactor model against recent PC bridge girder collision case study. The case study includes LiDAR scan information and photographs of visible damage.. In this study, the impactor model is evaluated with a primary focus to accurately predict residual deformation and observed damage with a sensitivity analysis of impact location, velocity and bed angle. These results afford the ability to develop pressure-impulse (P-I) statistical curves exclusive to the dump truck model and while the preliminary results are a representation of the collision analysis architecture, the analysis results are used to identify controlling bridge characteristics and investigate generalized P-I statistical curves and simplified impactor model analysis
52 – Today’s Science, Yesterday’s Past: Photogrammetric Mapping of Hickory Grove Missionary Church Cemetery in Chapel Hill, North Carolina
Barron Allison (NC Central University)
Accurately documenting cemeteries like the historic Hickory Grove Missionary Church Cemetery presents both challenges and opportunities. Traditional records, such as headstones and death notices, provide vital links to the past, but these often lack completeness due to missing markers, unmarked graves, and natural reforestation. By utilizing advanced technologies like Uncrewed Aerial Systems (UAV) and photogrammetry, we aim to capture high-resolution imagery to create precise spatial products that honor and preserve these sites. This project, a collaboration between North Carolina Central University’s Department of Environmental, Earth, and Geospatial Sciences (DEEGS) and Department of History, focuses on generating photogrammetric models from drone-captured imagery. These models support historical research by integrating data layers, including GPS and GIS-based cemetery records, which can indicate potential unmarked graves. The results offer a framework for preservation and potential use in planning, environmental justice, and cultural heritage initiatives.
53 – Assessment of the Aging Sensitivity of High Reclaimed Asphalt Pavement Asphalt Mixtures with Recycling Agents
Emilio Turbay (NC State University)
This study investigates the use of various recycling agents (RAs) in-lieu of using soft virgin binders to improve the cracking and long-term aging resistance of asphalt mixtures with high Reclaimed Asphalt Pavement (RAP) content. Two RAP sources and five RAs were evaluated using Short-Term Oven Aging (STOA) and a 1-day, 95°C Long-Term Oven Aging (LTOA) protocols. Performance was measured through the Cracking Tolerance Index (CTIndex) at the mixture scale and Glover-Rowe Parameter (GRP) at the binder scale. The results showed that the RA modified mixtures yielded equal or better CTIndex values at both age levels and associated aging sensitivity compared to the mixtures with soft virgin binders. However, the CTIndex values varied with the mixture source, RA type, and dosage in many cases. A mixture source-specific negative correlation between GRP and CTIndex was observed, confirming the importance of binder properties on mixture cracking resistance. However, given that the mixture source also had a strong impact, this study underscores the importance of LTOA protocols in Balanced Mix Design (BMD) procedures for reliable performance evaluation.
54 – Scooting After Sundown: Understanding Nighttime Usage Patterns and Regulatory Impacts
Lydia Adjetey (UNC Chapel Hill)
E-scooters have emerged as a popular mode of active travel, perceived as an environmentally friendly transport solution. The benefits of e-scooters include reduced congestion and air pollution. A study by NACTO (2019) revealed that shared e-scooter trips in 2019 doubled compared to 2018 and accounted for two thirds of all shared micromobility trips in the US. This trend has ignited academic and government interest in understanding the usage pattern of e-scooter users across the country. Largely, e-scooters are used for commuting, leisure trips, shopping related trips, and errand trips (Hardt et al., 2019). Despite the significant contribution of past research on users’ travel behavior, a gap remains in understanding how bans placed on e-scooter usage at night impact users who heavily rely on them for trips. This study seeks to ascertain nighttime e-scooter use, and the impact of nighttime bans on e-scooter use by analyzing a survey of e-scooter users in over twenty U.S. cities, including nine cities with nighttime e-scooter bans. Preliminary findings indicate that riders generally feel safe (85%) using e-scooters at night. This is reflected in the leading e-scooter trips of commuting to/from school (38%) and attending social gatherings (48%). In the absence of e-scooters at night, users shift their transport modes to walking, taxi cabs/ ride hailing, and bus/ shuttles which they find relatively unsafe with scores of 19%, 45%, and 20% respectively.
55 – User’s Preference for Trip Planner Interface and Factors Influencing Sustainable Transport Choices in Rural and Urban Areas
Ridwan Tiamiyu and Dr. Venktesh Pandey (NC A&T University)
Promoting sustainable transportation modes requires understanding the factors influencing user behavior and preferences. The study aims to examine the determinants of transportation choices in rural and urban areas, focusing on behavioral and infrastructural factors, and assesses user preferences for trip planner interfaces. To achieve this, a survey was conducted to identify key insights into what influences individuals’ choices for sustainable transportation. Results highlight strong support for trip chaining features, with 40% of respondents favoring the ability to plan multiple destinations and 41% preferring to select different modes for trip segments. Cost and travel time information emerged as top priorities, with 66% and over 50% of respondents, respectively, ranking them as essential criteria. The findings reveal significant disparities between urban and rural respondents. Urban participants were more receptive to sustainable transportation modes, while rural respondents identified poor infrastructure, limited public transit options, and longer travel distances as primary barriers. Across all demographics, practical information like avoiding congestion, road safety, and amenities such as gas stations and medical facilities were highly valued, while environmental data, such as carbon footprint, ranked lower in importance. The study emphasizes the importance of user-centric trip planner interfaces that prioritize cost, convenience, and safety to foster broader adoption of sustainable transportation. These insights emphasize the need for targeted infrastructure improvements, enhanced public awareness, and user-friendly digital tools to bridge the gap between urban and rural transportation needs and preferences. By addressing these challenges, this research provides actionable recommendations for promoting sustainable travel behavior.
56 – Mileage-Based User Fees: Addressing Equity and Behavioral Changes in North Carolina’s Transportation System
Rifa Tasnia (NC A&T University)
Our research explores mileage-based user fees (MBUF) as a sustainable solution to North Carolina’s declining motor fuel tax revenues, which are increasingly strained by the rise of fuel-efficient and electric vehicles. MBUF charges drivers based on miles traveled, offering an equitable distribution of infrastructure costs across geographic and demographic groups while supporting the state’s long-term transportation funding goals. The empirical data for this paper is obtained through “SUMTC” in April, 2024, in the North Carolina State. Our study focuses on three key objectives: (1) analyzing behavioral changes in individual and freight travel under varying MBUF rates, such as reduced travel demand, increased carpooling, shifts in departure times, and higher adoption of fuel-efficient vehicles; (2) assessing MBUF’s equity implications to ensure a fair distribution of cost burdens. A SP-survey and discrete choice modeling were used to assess travel behavior and mode preferences under hypothetical MBUF scenarios. Results show that 58% of respondents are willing to adopt MBUF if per-trip cost increases remain minimal. Urban respondents prioritize shorter travel times, while rural travelers value cost savings. Younger individuals are more MBUF-sensitive, while older adults are less likely to shift modes. Analysis on large-scale networks reveal that differential rates encourage higher-shifts to transit, carpooling, or park-and-ride options, show some reduction in congestion and emissions in urban areas by encouraging shifts to transit, carpooling, or park-and-ride options. Findings provide actionable-insights for the NCDOT to design equitable MBUF policies, implement effective pilot-programs, and support resilient infrastructure funding while addressing future transportation challenges.
57 – Equitable Flood Impact Analysis Modeling Transportation and Water Infrastructure Interoperability
Rifa Tasnia (NC A&T University)
Flooding poses a significant threat to transportation infrastructure, yet research on the effects of dynamic flood levels on road networks remains limited. Smart cities can leverage digital data on travel demand, infrastructure, and water levels to improve integrated flood management and promote social equity. This approach could specifically benefit vulnerable populations without vehicle access. Traditional traffic models do not consider terrain and elevation, which limits their ability to assess flood risks during a disaster. For state-level planning, this omission affects resource allocation and also long-term resilience strategies. The NCDOT Resiliency Report highlights the importance of resilient transportation infrastructures. Our study aims to capture the intertwined nature between water and transportation infrastructures, evaluating flood risks and their impact on road networks. We develop an interoperable digital twin framework that integrates road networks with simulations of rising water levels, focusing on rerouting strategies during floods to assess infrastructure vulnerabilities in both Hyde County, NC (a rural area) and Wilmington, NC (an urban area). Using tools like SUMO, Python, and QGIS, alongside elevation and HAND datasets, we simulate flooding scenarios to identify high-risk roads and intersections. Our findings indicate that (a) open-source tools can capture the impacts of rising water levels in identifying submerged roads, which show up as transverse or longitudinal lane closure in traffic simulators, enabling dynamic rerouting decisions, and (b) interoperable flood and water infrastructure models can enable prompt identification of at-risk roads and mitigate flash flooding events. Our findings can help facilitate better disaster preparedness and response strategies.
58 – Environmental Monitoring to Support Revised Steel Pile Corrosion Protection Specifications
Nazmuz Sakib (NC State University)
Premature corrosion of steel piles supporting bridges in North Carolina has been observed in many sites across the state, which may lead to significant maintenance costs and reduced structural longevity of these structures. Protective coatings have been observed to fail within 20 years, far earlier than anticipated. This research project, conducted in collaboration with NCDOT, aims to address this issue by developing a data-driven framework to improve corrosion protection strategies. The study includes comprehensive environmental monitoring at bridge sites prone to corrosion as well as control sites with minimal corrosion to compare conditions. A field-deployable monitoring system has been developed, integrating pH, dissolved oxygen, electrical conductivity, temperature, turbidity, and water depth sensors. Powered by solar energy and equipped with GSM communication for real-time data transmission, the system collects continuous data on factors affecting corrosion. Additional field sampling provides detailed lab analysis of parameters such as chloride and sulfate concentrations, establishing correlations with sensor data. A literature review will examine the effectiveness of existing protective coatings and include a comparison of standard corrosion prevention guidelines across different states. The project will use the collected data to identify critical environmental factors contributing to accelerated corrosion and recommend revised coating specifications tailored to site-specific conditions. This research will result in actionable insights for NCDOT, including site classification criteria, updated protective policies, and cost-effective solutions for extending steel pile service life. By addressing this critical infrastructure challenge, the project aims to enhance the durability and safety of bridges statewide.
59 – Performance evaluation of a Freeway segment using microscopic simulation
Md Abdullah Al Hasan (NC State University)
This study aims to evaluate the performance of a freeway segment in Portsmouth, Virginia, using TransModeler 7.0. The primary focus is to assess whether the freeway operates efficiently, analyzing key performance metrics. We selected the weaving segments to understand the challenges faced by drivers in decision-making for gap selection, which often lead to safety critical scenarios and increased travel times. We modified the existing Portsmouth simulation model by incorporating additional sensors at the freeway segments to capture dynamic flow patterns (speed, travel time, headway, lane changing events). Simulations were segmented into 15-minute time intervals for peak hours. We conducted a headway analysis which gives the estimation of the saturation flow rate. Additionally, we determined the cumulative distribution function (CDF) of travel times and identified their distribution through percentile values to identify slow and fast-moving vehicles. Our analysis also includes speed evaluation, lane-changing behavior, and a detailed examination of weaving actions within critical segments. The research outcome will provide insights into driver behavior in weaving sections, congestion management and overall network connectivity. In future, the policymakers can implement the improvement techniques such as VSL, ramp metering, etc.
60 – Enhancing Efficiency and Service Quality at License Plate Agencies in North Carolina
Mohammad Ashraf Ali (NC A&T University)
License Plate Agencies (LPAs) in North Carolina are vital for DMV-related services like titling and registration but face challenges in customer satisfaction and business profit in the existing transaction-based compensation model. This research, part of the ongoing RP2025-12 project funded by NCDOT, aims to address these issues by creating tiered, data-driven compensation models focused on customer service, financial viability, and technological alignment. Key findings from the review of LPA-related literature and compensation models from other domains highlight the need for performance-based contracts with metrics like customer satisfaction and error rates. Recommendations include system upgrades, self-service kiosks, and online service promotion to improve efficiency and reduce wait times. Research across other service industries shows that tailored compensation strategies, such as practical discounts or experiential rewards, align compensation with service expectations and ensure operational continuity. Furthermore, data-driven analysis reveals that complaints are uncorrelated to the transaction volume handled by the LPA and are likely governed by factors such as service quality, employee behavior, and facility accessibility, emphasizing the role of staff training, workflow optimization, and infrastructure improvements in enhancing customer satisfaction. State comparisons reveal diverse fee structures, such as Florida’s fixed fees and Pennsylvania’s market-driven rates. Texas and Louisiana rely on private providers, using convenience fees to enhance customer experience, with regulatory frameworks ensuring accountability. This ongoing study clarifies issues surrounding LPA operations, service obstacles, customer complaints, and areas for improvement. The findings aim to enhance customer satisfaction, support future contract designs, and provide a model for similar systems nationwide.
61 – Utilization of qualitative interview findings to mobilize community members and decision-makers to implement evidence-based cross-sector Safe Systems interventions
Audrey Payne (UNC Chapel Hill)
Despite declines in fatal traffic injury over the last few decades, surveillance data indicate that progress has stalled in recent years, even rising since 2022 in NC and across the nation. For the most vulnerable road users (pedestrians, cyclists), fatal crashes are on a precipitous rise. Significant racial and socioeconomic inequities exist in serious and fatal road injury with American Indian and Alaskan Natives experiencing more than twice the fatality rate compared to the general population, for example. In response to this issue, UNC Injury Prevention Research Center has adopted Vision Zero (VZ), a strategy to eliminate all traffic deaths and severe injuries, while increasing safe, healthy, equitable mobility for all persons. Though there is a rise in popularity of VZ in the US, there is still a need to address gaps and limitations to realizing VZ in communities. This project utilizes interview findings to create infographics that will mobilize community members with a vested interest in road safety (e.g. government leaders, constituents, etc.) about interventions that integrate the Safe Systems Approach (SSA) and advance the mission of VZ. Infographics are informed by interviews conducted with SSA and VZ thought leaders, implementers, and communication professionals providing perspectives on priority issues, successful interventions, and common themes related to transportation safety. This presentation reviews findings from the interviews and how the analysis process will inform the creation of infographics to be used to mobilize decision-makers on what interventions can be implemented to address the concerns of the communities they serve.
62 – Enhancing Traffic Safety and Efficiency in North Carolina: A Framework for Optimized Truck Parking and Reservation Systems
Komal Gulati (NC A&T University)
Truck parking shortages along North Carolina’s critical interstates—including I-26, I-77, I-85, and I-95—have resulted in illegal parking on freeway shoulders and interchange ramps, creating serious safety hazards and operational challenges. To address these issues, this research identifies and prioritizes candidate locations for additional truck parking along these corridors, solved as a facility location problem using geospatial prioritization. The prioritization framework evaluates parking size, safety metrics (number and type of crashes), proximity to amenities, detour times from existing facilities, and potential parking capacity for both short-haul and long-haul vehicles. These factors ensure selected sites meet trucker needs while enhancing regional safety. Building on these findings, budget scenarios are analyzed to optimize resource allocation. Under a high-budget scenario ($7.5M per lot), 19 of 519 state-owned parcels are shortlisted, while under a low-budget scenario ($3.5M per lot), 49 locations are selected. These optimized solutions maximize coverage and cost efficiency, addressing 90% of the unmet demand for additional truck parking capacity. To further improve parking efficiency and safety, a parking reservation model is developed as a complement to the facility location framework. This model integrates a Markov Decision Process (MDP) to simulate truckers’ decision-making processes and a Mixed-Integer Linear Programming (MILP) model to optimize parking allocation and minimize costs. Reinforcement learning techniques enable the system to dynamically adapt to fluctuating demand and operational constraints. These findings provide policymakers with actionable strategies to reduce illegal parking, enhance safety, and improve freight mobility across North Carolina.